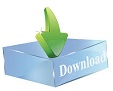

Quiver achieves up to 35$\times$ lower latency with a 8$\times$ higher throughput compared to state-of-the-art GNN approaches (DGL and PyG).īelow is a figure that describes a benchmark that evaluates the performance of Quiver in serving situation, PyG (2.0.3) and DGL (1.0.2) on a 2-GPU server that runs the Reddit with GraphSage. Quiver uses this metric to assign sampling tasks to GPUs only when the performance gains surpass CPU-based sampling and (2) for feature aggregation, Quiver relies on the feature access probability to decide which features to partition and replicate across a distributed GPU NUMA topology.

Quiver's key idea is to exploit workload metrics for predicting the irregular computation of GNN requests, and governing the use of GPUs for graph sampling and feature aggregation: (1) for graph sampling, Quiver calculates the probabilistic sampled graph size, a metric that predicts the degree of parallelism in graph sampling.
#Quiver reddit high five full#
result_queue_list()Ī full example using Quiver to serve a GNN model with Reddit dataset on a single machine can be found here. # result_queue_list = result_queue_list = server. # Instantiate the inference server component server = InferenceServer( model_path, dataset, sampled_queue_list. # sampled_request_queue_list = sampled_queue_list = hybrid_sampler. # Instantiate the sampler component hybrid_sampler = HybridSampler( dataset, batched_queue_list. # batched_request_queue_list = batched_queue_list = request_batcher. # Instantiate the auto batch component request_batcher = RequestBatcher( stream_input_queue. multiprocessing import Queue from quiver import AutoBatch, ServingSampler, ServerInference # Define dataset and sampler dataset = Reddit(.)
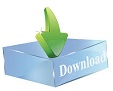